5 Questions for ... Pascal Friederich
Predicting new materials with AI
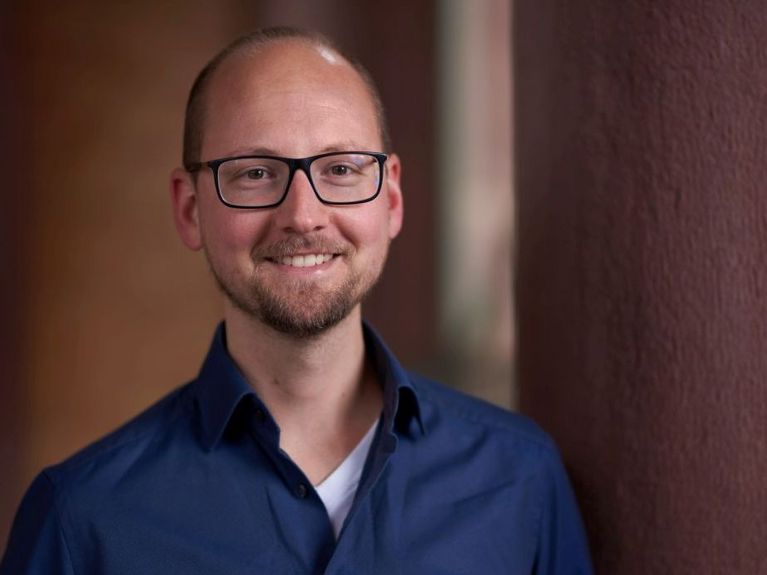
Pascal Friederich, professor at KIT, receives a Heinz Maier-Leibnitz Prize of the DFG. (Credit: KIT, Amadeus Bramsiepe)
Pascal Friederich from the Karlsruhe Institute of Technology (KIT) uses artificial intelligence to create new materials. For this, he and his team were awarded the Heinz Maier Leibnitz Prize of the German Research Foundation (DFG).
Mr. Friederich, the German Research Foundation is awarding you the Heinz Maier-Leibnitz Prize for your research on the use of artificial intelligence in materials research. How can algorithms help develop new materials?
The properties of new materials can be simulated on the computer without the materials even existing yet. This mainly involves simulations of the quantum mechanics that underlie material properties. A nice example here comes from organic electronics. How a particular material interacts with light at the quantum level determines its capabilities as an organic solar cell or display. Calculating something like this, however, is very complex, lengthy and expensive. It requires a lot of computer power and computing time on mainframe computers. With machine learning methods, this effort can be reduced. This is because AI can predict the properties of several million materials from the results of perhaps 1,000 material simulations. In doing so, we vary not only the composition of the materials, but often also the processing conditions. This is because a material is not only defined by what elements it is made of. The way it is manufactured also has a significant influence on its properties. The goal here is always to find new material candidates that can then be manufactured in the laboratory and hopefully confirm what we have predicted. And even if we don't have simulation methods for a material, AI can help. We can have the algorithm learn in automated experiments, for example, so that the AI then suggests the next experiment. With all these methods, we want to gain a speed advantage by reducing the development time for new materials from the usual 20 years to maybe ten or even five. This is an enormous advantage for the further development of essential technologies. And that speed advantage can be provided precisely by AI methods.
What led you to combine computer science and materials science?
I studied physics and worked on the simulation of organic electronics in my master's thesis. At that time, simulations were still very common tools to confirm experiments that had already been done; to understand them; and to streamline them a bit. Even during my master's thesis, and then even more during my PhD, there was this trend of speeding up simulations to the point where they were one step ahead of the experiments. So at that time we could already test thousands of materials on the computer and give tips to the experimenters. However, you very quickly reach the performance limits of the computers. At the same time, there were also more and more successes in the machine learning area, for example for computer vision, for machine translation, actually for everything for which AI is already used today in everyday life. And there were first approaches to combine simulations with AI. That's exactly what I did in my post doc time. I went to the US on a Marie Sklodowska-Curie grant to join a research group at Harvard University that had pioneered work in this area. I moved to Toronto with this group in 2018. We worked intensively on how to link simulation methods with AI. And then I got the call back to Karlsruhe to continue working on this here.
What particularly fascinates you about your work?
I find it particularly fascinating that we can make statements about materials that no one has ever made before. More and more often, we get the chance to get ahead of the experiment with the help of computer simulations and data-driven models. In particle physics, something like this has been around for a long time. Particles are predicted based on theories and experimentally confirmed years or decades later with huge accelerators. In chemistry and materials science, however, this is only just emerging. And being able to witness this tipping point towards virtual material development is something I find extremely fascinating. Certainly, AI methods are only one tool in this process, but a very exciting one. I don't think we can even estimate where the limits of AI methods are at the moment.
Materials development is now a huge field of activity. In which direction are you personally drawn?
Materials that can generate green energy or increase energy efficiency have always been with me in my research. These are the classes of materials that also motivate me personally. Because with these I see a big impact, especially when it comes to societal challenges like the energy transition. That's what drives me, and here I also see a deeper sense of wanting to develop new materials more quickly in order to solve urgent problems. That's not the only topic we specialize in, but it's a common thread that has run through the years.
What does the Heinz Maier-Leibnitz Prize mean for you personally and for your scientific career?
First of all, the prize generates a lot of attention - not only inside but also outside KIT and will certainly open some doors. Personally, the prize motivates me to continue as before. Because the prize shows that my science is considered relevant and that the scientific community benefits from it. Therefore, the win is also a great motivational boost for me.
Readers comments