Human Radiome Project
"ChatGPT for Medical Professionals"
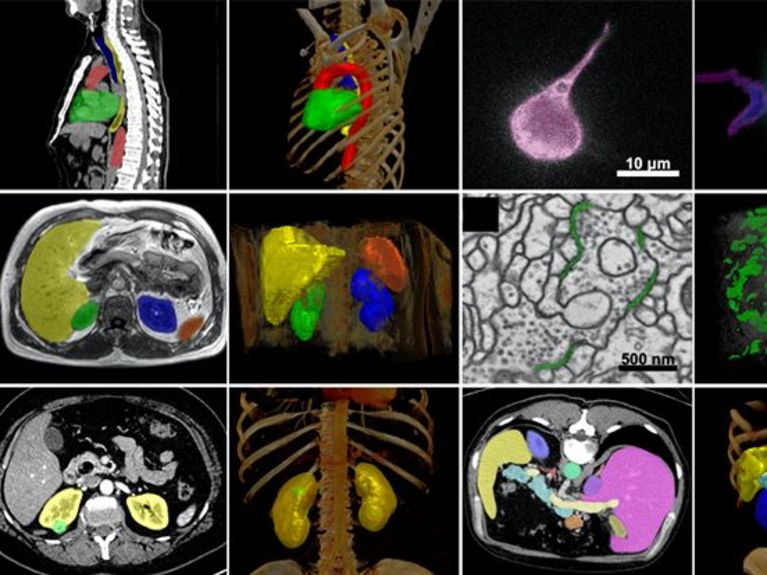
Picture: Fabian Isensee / DKFZ
What does an artificial intelligence (AI) model look like that can answer a wide variety of medical questions using radiological image data? The Human Radiome Project aims to set the gold standard.
Doctors use images from MRIs and CT scans to detect and monitor many conditions, including narrowed heart vessels, brain tumors, and Alzheimer's disease. AI models have long played a role in processing this growing volume of images. As of March 2024, 76% of all approved AI applications in the U.S. were radiology applications.1
An AI model is asked to perform a very specific task, such as detecting brain tumors. For this task, it is given as much high-quality training data as possible. The training data forms the basis of the AI's functionality and must be annotated by experts down to the smallest detail. This not only takes a lot of time and money, but the image data is often not available in the required quantity and quality.
The research team led by Paul Jäger, Klaus Maier-Hein, Fabian Isensee and Lena Maier-Hein at the German Cancer Research Center (DKFZ) in Heidelberg is now breaking new ground with the Human Radiome Project: They are training a basic radiological AI model, i.e., a model with broad basic knowledge. The most well known basic model is probably Chat GPT, however, the chat bot is based on human language and not on radiological images.
Once a model is trained in a basic way, specializations are then possible. "With the Human Radiome Project, we in Germany are laying the foundation for something that is just getting started," says Klaus Maier-Hein.
We want to make difficult tasks easier for doctors, and we are providing well-functioning tools to do so.
The training data for the Foundation model does not need to be annotated as the model learns to recognize structures in the images itself, becoming a kind of imaging super-brain (see box). However, this requires a lot of data. The Human Radiome Project team can draw from a particularly rich pool: Its basic model is pre-trained with more than 4.8 million 3D image data points from MRI, CT and PET. With 40 times more data than previously available models2, it contains the world's largest radiological 3D image dataset. "This is also thanks to our excellent network," says Klaus Maier-Hein. In addition to the quantity of images, 3D is also important because it is the best way to assess anatomy and pathology. The 3D image data come from the DKFZ and partner universities in Bonn, Heidelberg and Basel, as well as from various publicly available data sets. "The more and more diverse the training data, the better the performance of the AI in the real world," says Klaus Maier-Hein.
The basic model can be used to train specialized AI for more than 70 different tasks on the broad data base. This requires only a small amount of carefully selected and annotated data. Among other things, the Foundation Model will learn to analyze neurological diseases, segment brain tumors and determine cancer risks. Industry partners Siemens Healthineers and FLOY GmbH, and other Helmholtz Association partners such as the German Center for Neurodegenerative Diseases (DZNE) and the Max Delbrück Center for Molecular Medicine (MDC) will provide additional data. Later, users can use the pre-trained model to adapt it to their specific research question with a small amount of their own data.
The Helmholtz researchers are making their Foundation model available as an open source platform. "We want to benefit the community and increase the visibility of science", says Klaus Maier-Hein. He hopes that different users, including start-ups, will use the model to develop solutions for specific medical applications. Commercialization is not the team's focus, but later applications are welcome to earn money based on Helmholtz-funded research.
The DKFZ researchers have already achieved outstanding research results with AI models3. Their most recent AI-based biomedical image analysis method won more than 30 international competitions and enjoys high international recognition. The new model will also demonstrate its capabilities in public competitions, such as those organized by the Medical Image Analysis Competitions (MICCAI).
Clinical applications: What will be possible? What is important?
The Human Radiome Project will help improve radiological diagnostics and medical therapy: Assessing cancer progression under therapy, detecting rare diseases, finding hard-to-detect abnormalities. This is because the model also recognizes data patterns that are virtually invisible to humans. They can provide additional information and indicate disease.
An AI model looks at an MRI or CT image with an open mind: It "sees" more than just the organ being examined. For example, MRI images of the breast, such as those taken for breast cancer screening, also show the aorta. However, only the breast is evaluated by a human, because it is a breast cancer screening. An AI model can specialize in both the breast and the aorta. "The AI recognizes abnormalities in the aorta more reliably than a radiologist," says Klaus Maier-Hein.Useful disease characteristics
The AI is also faster and more accurate than previous methods at estimating tumor size. It can detect tumor boundaries in 3D to calculate tumor volume, so multiple scans over time can provide accurate information about how quickly a tumor is growing, or shrinking under treatment.
The example of tumors illustrates how important it is for IT and medicine to work together to train AI models correctly. After all, it's not just about size. "We need to know what is important for doctors and patients, and that includes not only the growth of a brain tumor, but also the question of whether there are new foci, for example", says Klaus Maier-Hein. This is exactly what the team has just published: Which features are helpful and crucial for clinical applications?
If the AI measures a brain tumor 0.1 percent more accurately than the competition, this may be statistically good, but it misses the clinical question. This is because the exact diameter of the main tumor is less decisive for the patient's therapy than the existence of a new tumor focus, no matter how small it may be.
AI developers like Paul Jäger, Klaus Maier-Hein, Fabian Isensee, Lena Maier-Hein and their team will change medicine together with artificial intelligence and put radiology ahead of other disciplines with the Human Radiome Project.
What does the Foundation Model of the Human Radiome Project learn?
Foundation models are trained using immense amounts of data. Until now, data for AI models had to be annotated at the pixel level. This had to be done manually, which requires expertise, time and money. An AI model built in this way is highly specialized and only suitable for very specific scenarios. Rare diseases could not be addressed at all due to the limited amount of data.
The Human Radiome Project's Foundation Model learns on the basis of deep learning without extensive manual annotations. It recognizes basic features and patterns from the images on its own (self-learning model) and invents its own annotations (pseudo-labels). The learning process includes, among other things, correctly supplementing images that intentionally lack information. The language model ChatGPT also learned this way: with texts and spaces that the algorithm was supposed to meaningfully supplement.
During pre-training, it is important that the images are of different quality. The AI model must be able to work with all data. If a model is trained only with high-quality data, it will not work with lower-quality data. “I can also teach a layperson to recognize brain tumors. However, the specialized knowledge learned no longer works as soon as the layperson sees images that were taken with a different system or different settings. They are unable to perform the transfer,” explains Klaus Maier-Hein. The pre-training of the DKFZ model can be roughly compared to the wealth of experience of a senior physician, who, based on their experience, can more easily perform transfer tasks.
After that, the foundation model can specialize in certain issues. For this fine-tuning, the model is given further, this time detailed annotated image data, as well as medical study data from the population and, in addition, research results.
The result: the representation, recognition and classification of a wide range of diseases and their development is possible. The AI model can support diagnoses, help with therapy planning, and monitor tumor growth in cancer patients – depending on what the fine-tuning is aimed at.
How much energy does the Human Radiome Project's foundation model need?
Learning the AI model's skills requires a lot of computing power: the 4.8 million 3D image data – MRI, CT and PET – correspond to over 500 terabytes (1 terabyte is roughly comparable to the data volume of 200 feature films, so 500 TB is the data volume of 100,000 feature films). An estimated 990,000 GPU (Graphics Processor Unit) hours and 4,000 CPU (Central Processing Unit) hours are planned for the image processing and machine learning process. The Human Radiome Project uses the JUWELS supercomputer in Jülich for this enormous computing power.
1 Website der US Food&Drug Administration/US-Behörde für für Lebens- und Arzneimittel: Artificial Intelligence and Machine Learning (AI/ML)-Enabled Medical Devices. Besucht am 15.07.2024
2 Wang G, Wu J, Luo X et al. MIS-FM: 3D Medical Image Segmentation using Foundation Models Pretrained on a Large-Scale Unannotated Dataset. arXiv:2306.16925
3 Isensee F, Jaeger PF, Kohl SAA et al. nnU-Net: a self-configuring method for deep learning-based biomedical image segmentation. Nat Methods. 2021 Feb;18(2):203-211. https://doi.org/10.1038/s41592-020-01008-z
4 Maier-Hein L, Reinke A, Godau P et al. Metrics reloaded: recommendations for image analysis validation. Nat Methods. 2024 Feb;21(2):195-212. doi.org/10.1038/s41592-023-02151-z
Helmholtz Foundation Model Initiative
Foundation models are a new generation of AI models that have a broad knowledge base and are therefore able to solve a range of complex problems. They are significantly more powerful and flexible than conventional AI models and therefore hold enormous potential for modern, data-driven science. They can become powerful tools that answer a wide range of research questions. The Helmholtz Association is ideally placed to develop such pioneering applications: a wealth of data, powerful supercomputers on which the models can be trained, and in-depth expertise in the field of artificial intelligence. Our goal is to develop foundation models across a broad spectrum of research fields that contribute to solving the big questions of our time.
Readers comments