Foundation Models
An AI model of the global carbon cycle
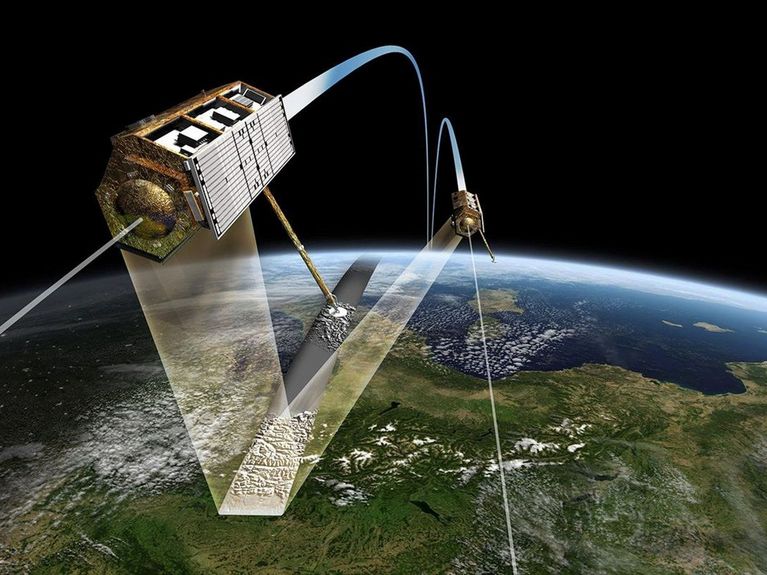
The data from TanDEM-X is also incorporated into the Foundation Model. Picture: DLR
A new basic AI model will help to better understand the global carbon cycle and make predictions about future changes. The project needs one thing above all else: Lots of high-quality data from multiple sources.
In early February, the Copernicus Climate Change Service reported that the average global surface temperature between February 2023 and January 2024 was 1.5 degrees Celsius above pre-industrial levels. The much quoted 1.5 degree target is a reality.
The primary cause of global warming is dramatic changes in the world's carbon stocks, particularly the burning of fossil fuels and the resulting release of carbon dioxide into the atmosphere. Climate change and human land use are also altering other natural carbon reservoirs. The interactions are complex, and the global carbon cycle is far from understood. "Only if we understand the global movement of carbon between reservoirs can we at least partially control our climate and effectively counteract the climate crisis," says Guido Große, geologist and permafrost expert at the Alfred Wegener Institute Helmholtz Centre for Polar and Marine Research (AWI) in Potsdam. Although we now know how much carbon is released by the consumption of fossil fuels, "the seasonal, regional and global changes in the very large reservoirs in vegetation and soils have not yet been sufficiently recorded for real carbon management. As a result, climate models currently do not take this into account accurately enough," says Große.
A three-year project led by Große as part of the Helmholtz Foundation Model Initiative aims to remedy this situation. Foundation models are special models in the field of artificial intelligence (AI) that are trained using large data sets. This broad but still relatively unspecific basic training enables AI models to recognize complex patterns and correlations on their own. This allows them to apply their extensive knowledge from training to many different tasks – so-called downstream tasks – for which they were not previously trained. For these tasks, they often need only a small amount of additional, specific data to work effectively. This is what distinguishes foundation models from traditional AI applications.
In permafrost soils, the amount of heat accounts for only nine percent of continental heat storage, but the increase in recent years has increased the thawing of permafrost and thus the release of greenhouse gases.(Picture: AWI / Julia Boike)
Under the name 3D Above and Below Ground Carbon Stocks (3D-ABC), Große and his team are developing a foundation model to capture and visualize the global terrestrial carbon budget, primarily from remote sensing data and large databases of biomass and soil carbon, as well as vegetation and soil carbon fluxes. From the tops of the tropical rainforests to the boreal forests of North America, from the European peat bogs to the deep permafrost soils of the Siberian tundra, the AI-based base model will initially use the vast amounts of data to capture the large terrestrial carbon reservoirs. Satellite imagery and laser data will be incorporated into the model, while multicopters and ground sensors have been providing additional relevant information for years. What's more, the resolution and power of remote sensing is increasing every year, and the observable periods are getting longer.
However, the amount of data is now too great for humans to handle alone. In addition to the AWI, the Jülich Research Centre, the German Research Centre for Geosciences, the Helmholtz Centre Dresden-Rossendorf, the Helmholtz Centre for Environmental Research and the German Aerospace Centre are also involved.
Große believes that research into permafrost soils is particularly important. After all, they are among the world's largest carbon reservoirs, currently storing around 1,600 billion tons of carbon which is almost twice as much as the atmosphere (880 billion tons). Because the Arctic is warming much faster than the global average, permafrost soils are particularly vulnerable to change. The erosion of riverbanks and coastlines, as well as the constant thawing of the landscape, exposes old, previously sequestered carbon to microbes, and ultimately to the atmosphere, exacerbating the climate crisis. Although this microbial release does not happen overnight, "it is not yet included in current climate models," says Große.
Prof. Dr Guido Grosse, Head of Permafrost Research Section at the Alfred Wegener Institute. Picture: AWI
"But documenting the current state is only the beginning of the Foundation Model," says the geologist. The key question is: How will these large carbon reservoirs change in the future, in a warmer world, with even more deforestation and more intensive agriculture in certain regions? It has long been known that many land reservoirs are subject to large annual carbon fluxes. In some regions, the flux is so large that some carbon sinks become large carbon sources, at least temporarily: Instead of absorbing carbon dioxide from the atmosphere, certain ecosystems release it in large quantities. "Here, too, no climate model is currently able to simulate the sometimes very rapid developments, such as changes in land use or deforestation of rainforests, which could intensify in the coming years and decades, reliably and in detail," says Große. Artificial intelligence, which builds on the wealth of scientific observation data from satellite missions and field studies, could help.
But it is precisely this kind of information – provided in a timely, high-resolution and generally understandable form – that is essential for policymakers and business to take effective measures for climate protection and adaptation: Which sinks do we need to protect more rigorously? What sources should we avoid?
This is exactly what 3D-ABC will do. Using harmonized data from the large Landsat and Sentinel-2 satellite missions and the German radar satellite mission TanDEM-X, the basic model will first be trained to understand and reproduce the global land cover and vegetation heights in the Amazon rainforest, tundra and taiga as accurately as possible. What grows where, how high and how dense? With this information, the model will learn to determine biomass and draw detailed conclusions about the carbon budget above and below ground. Remote sensing data, for example, from satellites and drones, also record changes in the land surface. Large fires, the expansion of infrastructure, erosion or insect infestations can cause major damage to vegetation within a few days, weeks or months. This in turn affects local carbon stores. The final phase of the project will focus on carbon fluxes. "We hope to be able to train the model not only to reflect the reservoirs correctly, but also to predict carbon fluxes in high spatial and temporal detail," says Große, describing the central challenge that would be essential for actual carbon management on a global scale.
Another hurdle, according to Große, is the storage of the huge amounts of data. The information is processed at the Helmholtz Association's own supercomputer center in Jülich. However, the long-term storage capacity there is limited. A solution is currently being sought. Everyone involved in the project agrees that the potential and possible benefits of 3D-ABC are enormous. But so are the challenges that lie ahead. "To achieve the desired effect," says Große, "we have to make sure that the foundation model can work with a wide variety of imagery. With optical and radar satellite data, and with 3D information from laser measurements. If we can achieve this multimodality, we will have created an excellent tool for global monitoring of terrestrial carbon.
Helmholtz Foundation Model Initiative
Foundation models are a new generation of AI models that have a broad knowledge base and are therefore able to solve a range of complex problems. They are significantly more powerful and flexible than conventional AI models and therefore hold enormous potential for modern, data-driven science. They can become powerful tools that answer a wide range of research questions. The Helmholtz Association is ideally placed to develop such pioneering applications: a wealth of data, powerful supercomputers on which the models can be trained, and in-depth expertise in the field of artificial intelligence. Our goal is to develop foundation models across a broad spectrum of research fields that contribute to solving the big questions of our time.
Readers comments